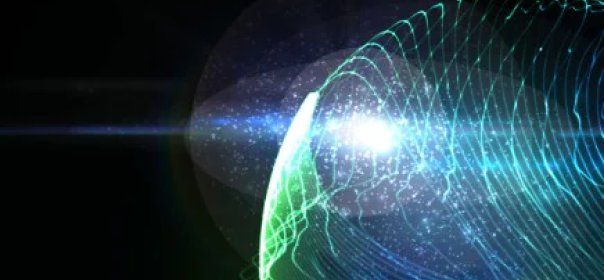

Data is something which can be derived not just from structured data sources but from twitter feeds, and various other sources that are unstructured. Data finds a new meaning when you then apply cloud computing to it. With the cloud, companies are free of the dilemma of choosing what to retain and store, and what to not. Organizations want to leverage data to fuel innovation and convert it for business advantage.
- Extends or evolves the Data Warehouse (DW) architecture.
- It provides the capability to store any data in any format.
- Secure, compliant, and auditable.
- Runs any type of analytics from DW to predictive.
From moving data and getting it on the cloud from anywhere, there are a bunch of services that pave a way to process and protect your data. The AWS analytics portfolio covers the data journey from discovery to migration to the cloud. Once the data is on the cloud, there are several ways to archive the old data and aggregate existing data. A structured data analysis, serverless query, and search can also be integrated with the application to get insights from the data.
Artificial Intelligence is the field of computer science that mimics a machine to be a human brain, while ML is a subset of AI, where the machine learns on its own and performs tasks automatically. Deep learning is a subset of ML. If we were to compare it to the human brain, it is similar to neurons that are connected and trying to figure out patterns. These neural network algorithms can further dive deep and classify data. ML is a subset of AI and Deep Learning is a subset of ML.
Amazon has been using machine learning across the company for the past 20 years. For example, if you buy a product on Amazon, it will recommend similar products to you. These recommendations are powered by AI. So, why is everyone talking about AI/ML now?
More than a decade ago, the computational power and storage space required to do ML project was unavailable. The skilled professionals required were in limited supply, and the costs to run ML workloads were prohibitive. Today, with cloud computing, access to the infrastructure required is more pervasive, and the cost of doing ML has reduced significantly, making it more feasible to implement.
Today a developer in a small organization, can build, train, and deploy a model, and then derive inferences out of it. Today, although there has been a multi-fold growth in data, the computational time to process machine learning models has significantly reduced. AWS’s vision is to put machine learning in the hands of every developer
To quote Andy Jassy, CEO of AWS, “Invention requires two things: the ability to try lots of experiments, and not having to live with the collateral damage of failure.” With cloud analytics, the costs have come down significantly. You can pay for what you use, experiment a lot, fail fast, which ultimately leads to innovation. This helps companies grow and gives them a much- needed competitive advantage.