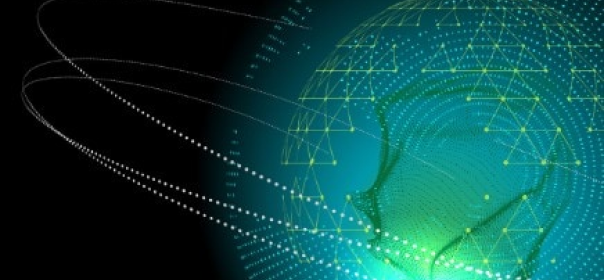

While several businesses today focus on finding ways to effectively derive value from data with the help of which they desire to acquire certain business outcomes, doing so would be no cakewalk. Mining data for the purpose of carving analytics applications with the aim of driving competent decision-making and innovation takes a lot of expertise and resources. To make this process smoother and more efficient, businesses are choosing to replace the traditional data management with a gradually emerging set of practices known as DataOps.
DataOps has the potential to have incredible transformative effects on data processes. Its key aim lies in enabling companies to implement a process that effectively manages and uses their ever-increasing data stores in a competent fashion, ultimately reducing the overall time of data analytics.
While DataOps has not yet become mainstream when it comes to building data solutions, it essentially has raised a great amount of interest among people following the market evolution actively. In 2019 Gartner estimated the adoption rate of DataOps as less than 1% of the addressable market. Even though this is a relatively new domain, various proven technologies and competent practices involved in it are gradually becoming quite popular. These practices are known to possess expansive applicability for operational agility, data governance, as well as data analytics. DataOps is considered to be especially suitable for driving ML, AI, and deep learning methods, as these data-hungry technologies majorly require progressive improvements for the purpose of remaining effective.
DevOps has transformed the way how applications are built in the industry by applying agile practices to the process of product development and testing, and in a similar fashion, DataOps is concentrated on revolutionizing how data is integrated, shared and made available to the people.
In addition to aiding company managers to improve the accuracy and speed of their business insights with the help of automation analytics-ready data pipelines, the practice of DataOps would also eventually transform how data is consumed across a firm as a whole. This solution would ideally break down the silos in IT operations, ultimately paving the way to build superior speed and accuracy of data analytics. By efficiently leveraging real-time integration technologies, like CDC or change data capture as an element of its overall set of principles, DataOps is known to be expected to disrupt and eventually transform the data processes prevalent across the industry.
The new cultural practices, solutions, and processes that are at the core of DataOps tend to allow firms to eliminate the manual processes that are associated with data delivery, making the system more agile and secure. These processes majorly include data versioning, provisioning and aligning database code with the relevant application code. DataOps subsequently helps in the fast provisioning of data production with masked data, while also synchronizing the database schema.
In the coming years, it is expected that more and more companies would educate themselves on the practice of DataOps, as it would gradually start its transition journey from an abstract idea to a tangible practice. In the future, one might expect to see this practice further revolutionize the usage of information in the data era.